Quantum Machine Learning: Algorithms of Tomorrow
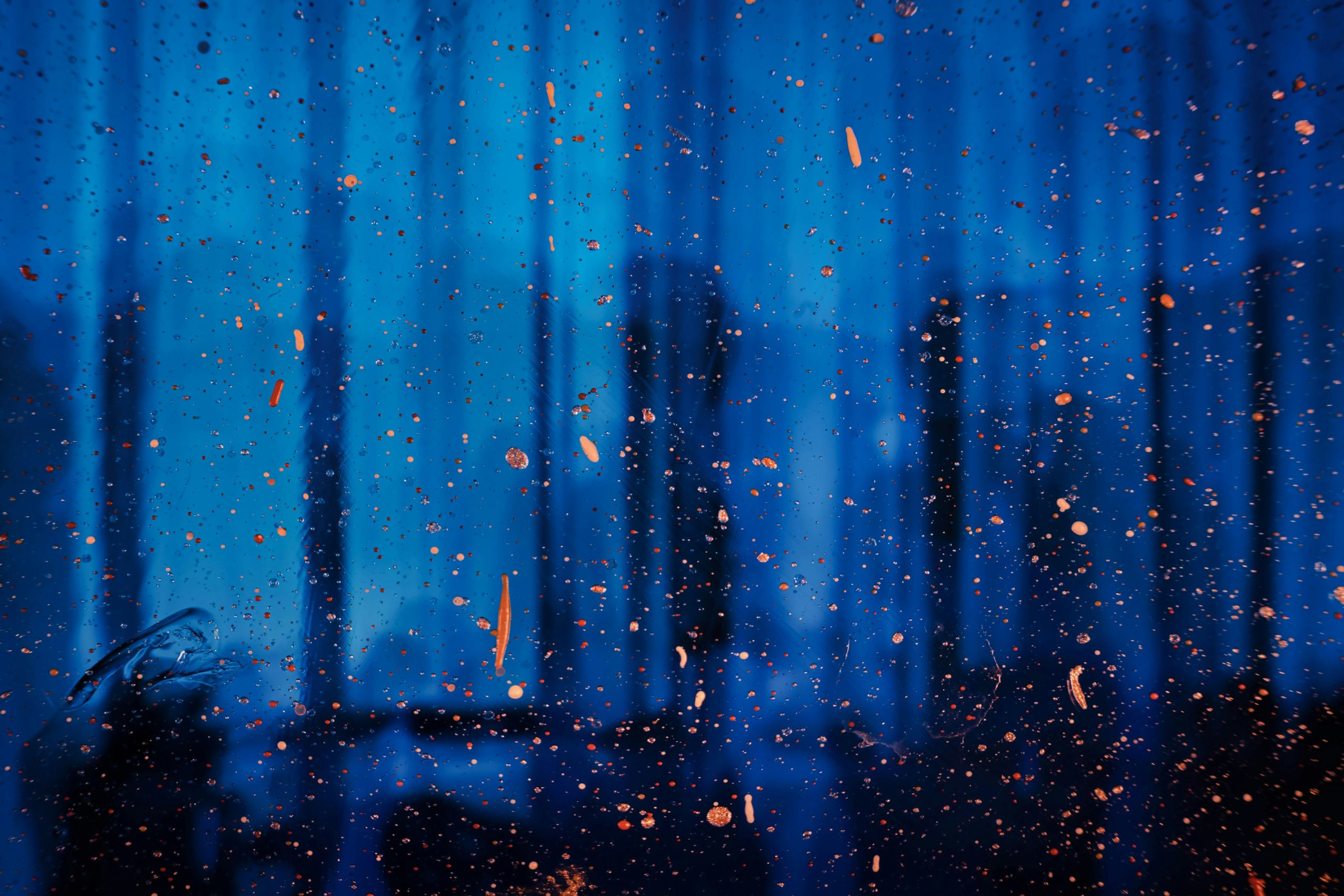
Before diving in, please note: This post is for informational purposes only. If you’d like to know more about how we approach topics, feel free to check out our friendly Disclaimer Page.
Hey there, amazing readers! 🖐️ Just a quick note: yes, we know there are a lot of ads here. Trust us, we get it—it’s not the prettiest look, but they help us keep this blog alive and kicking. Those pesky little ads cover the costs of all the behind-the-scenes magic, from hosting and tech stuff to creating content we hope you’ll love.
We’re committed to delivering quality posts, and your support (even just sticking around despite the ads) means everything to us. So, bear with us, and thanks for helping us keep the good vibes rolling. Now, on to the fun stuff! 😉
TRANSLATE BUTTON AT THE END OF THE ARTICLE
A Quick Overview
In the realm of cutting-edge technologies, Quantum Machine Learning (QML) stands at the forefront, combining the power of quantum computing with the capabilities of machine learning algorithms.
This innovative field is poised to revolutionize how we process and analyze complex data sets, unlocking new possibilities for solving intricate problems that classical computers struggle to address.
By harnessing the principles of quantum mechanics, QML holds the promise of exponentially speeding up computations and providing more accurate results.
Introduction to Quantum Machine Learning
Quantum Machine Learning is a fusion of two groundbreaking fields: quantum computing and machine learning.
At its core, QML leverages quantum algorithms to enhance the speed and efficiency of traditional machine learning processes.
By utilizing quantum bits, or qubits, which can exist in multiple states simultaneously, QML algorithms can explore vast solution spaces and evaluate multiple possibilities in parallel.
This parallelism allows for the rapid optimization of complex mathematical functions, making quantum machine learning a game-changer in the world of data analysis.
Understanding Quantum Computing Basics
Quantum computing operates on the principles of superposition and entanglement, allowing quantum bits to exist in multiple states at once and exhibit correlations with other qubits, respectively.
These properties enable quantum computers to perform calculations at unprecedented speeds for certain types of problems.
Unlike classical bits, which can only be in one of two states (0 or 1), qubits can represent a continuum of states between 0 and 1, leading to exponential computational power.
Quantum algorithms harness these quantum properties to solve complex problems efficiently.
Importance of Quantum Machine Learning
The significance of Quantum Machine Learning lies in its ability to tackle computationally intensive tasks that surpass the capabilities of classical computers.
From cryptography to optimization problems, QML offers a new paradigm for processing data and deriving insights.
By leveraging the unique properties of quantum mechanics, such as superposition and entanglement, quantum machine learning algorithms can provide more accurate predictions and faster solutions, paving the way for advancements in various industries.
Quantum Algorithms vs. Classical Algorithms
Quantum algorithms differ from classical algorithms in their approach to problem-solving.
While classical algorithms operate sequentially, quantum algorithms harness the power of superposition and entanglement to explore multiple solutions simultaneously.
This parallelism enables quantum algorithms to outperform classical algorithms for specific tasks, especially those involving large datasets or complex optimization problems.
Quantum machine learning algorithms excel in scenarios where classical algorithms struggle, offering a new frontier for innovation and discovery.
Advantages of Quantum Machine Learning
The advantages of Quantum Machine Learning are evident in its ability to process vast amounts of data at unprecedented speeds.
By leveraging quantum parallelism, QML algorithms can explore solution spaces exponentially faster than classical algorithms, leading to quicker insights and more accurate predictions.
Additionally, QML algorithms are well-suited for optimization tasks that require evaluating numerous possibilities, making them ideal for industries such as finance, healthcare, and logistics.
The potential for quantum machine learning to revolutionize data analysis is immense.
Challenges in Implementing Quantum ML
Despite its vast potential, Quantum Machine Learning faces several challenges in implementation.
One major hurdle is the current limitations of quantum hardware, which lacks the scalability and stability required for complex computations.
Additionally, developing quantum algorithms that can outperform classical algorithms for a wide range of problems remains a significant challenge.
Overcoming these obstacles will require continued research and investment in quantum computing technologies to realize the full potential of Quantum Machine Learning.
Current Applications of Quantum ML
While Quantum Machine Learning is still in its early stages, several industries are already exploring its potential applications.
In finance, QML algorithms can be used for portfolio optimization and risk management, while in healthcare, they can aid in drug discovery and personalized medicine.
Other fields, such as cybersecurity and logistics, are also beginning to adopt QML for solving complex optimization problems.
As quantum computing technology advances, the range of applications for Quantum Machine Learning is expected to grow significantly.
Future Prospects in Quantum Machine Learning
The future of Quantum Machine Learning holds immense promise for revolutionizing how we process and analyze data.
As quantum computing technology continues to advance, we can expect QML algorithms to become more sophisticated and capable of solving increasingly complex problems.
From quantum neural networks to quantum support vector machines, the possibilities for quantum machine learning are vast.
By pushing the boundaries of quantum computing and machine learning, researchers are paving the way for a new era of innovation and discovery.
Prominent Quantum Machine Learning Algorithms
Several prominent Quantum Machine Learning algorithms have emerged as frontrunners in the field.
These algorithms leverage the power of quantum parallelism to achieve faster and more accurate results than their classical counterparts.
Some notable QML algorithms include:
Quantum Boltzmann Machines: These algorithms use quantum annealing to optimize energy functions and perform probabilistic modeling.
Quantum Variational Algorithms: Based on variational methods, these algorithms aim to find the ground state of a quantum system through optimization.
Quantum Support Vector Machines: These algorithms leverage quantum parallelism to classify data points in high-dimensional spaces efficiently.
These algorithms represent just a glimpse of the potential for Quantum Machine Learning to revolutionize data analysis and decision-making processes.
Quantum Machine Learning in Various Industries
Quantum Machine Learning holds immense promise for various industries looking to enhance their data processing capabilities.
In finance, QML algorithms can optimize trading strategies and risk management models, leading to more profitable outcomes.
In healthcare, QML can aid in drug discovery and personalized treatment plans, improving patient outcomes.
Industries such as cybersecurity, logistics, and energy are also exploring the benefits of QML for solving complex optimization problems.
As quantum computing technology matures, the applications of Quantum Machine Learning will continue to expand across different sectors.
Quantum Machine Learning Research Trends
Researchers in the field of Quantum Machine Learning are actively exploring new algorithms and applications to push the boundaries of what is possible with quantum computing.
Some current research trends include:
Quantum Reinforcement Learning: Researchers are investigating how quantum algorithms can enhance reinforcement learning models to improve decision-making processes.
Quantum Generative Adversarial Networks (QGANs): This emerging area focuses on using quantum algorithms to generate new data samples for training machine learning models.
Quantum Transfer Learning: Scientists are exploring how quantum computing can facilitate transfer learning techniques to improve model generalization and performance.
These research trends highlight the dynamic and ever-evolving nature of Quantum Machine Learning, as researchers continue to unlock new capabilities and applications for this groundbreaking field.
Conclusion: The Growing Impact of QML
In conclusion, Quantum Machine Learning represents a paradigm shift in how we approach data analysis and problem-solving.
By harnessing the power of quantum computing, QML algorithms can process vast amounts of data at unprecedented speeds, leading to more accurate predictions and insights.
While the field of Quantum Machine Learning faces challenges in implementation, the potential for innovation and discovery is immense.
As industries across the globe begin to adopt QML for a wide range of applications, the impact of quantum machine learning is only set to grow in the coming years.
With ongoing research and advancements in quantum computing technology, Quantum Machine Learning is poised to revolutionize the way we interact with data and unlock new possibilities for the future.
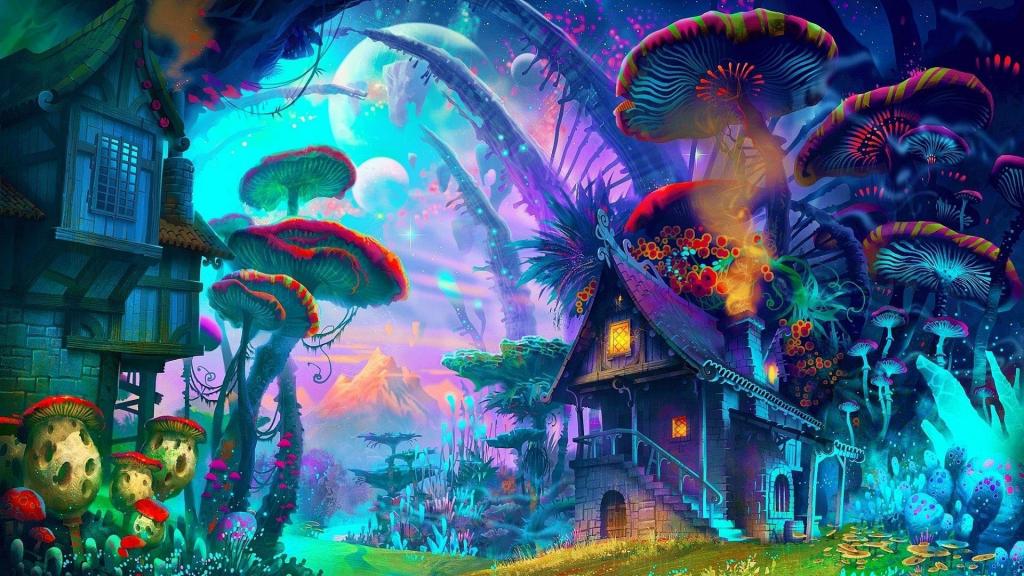
The Enlightenment Journey is a remarkable collection of writings authored by a distinguished group of experts in the fields of spirituality, new age, and esoteric knowledge.
This anthology features a diverse assembly of well-experienced authors who bring their profound insights and credible perspectives to the forefront.
Each contributor possesses a wealth of knowledge and wisdom, making them authorities in their respective domains.
Together, they offer readers a transformative journey into the realms of spiritual growth, self-discovery, and esoteric enlightenment.
The Enlightenment Journey is a testament to the collective expertise of these luminaries, providing readers with a rich tapestry of ideas and information to illuminate their spiritual path.
Our Diverse Expertise 🌟
While our primary focus is on spirituality and esotericism, we are equally passionate about exploring a wide range of other topics and niches 🌍📚. Our experienced team is dedicated to delivering high-quality, informative content across various subjects ✨.
To ensure we provide the most accurate and valuable insights, we collaborate with trusted experts in their respective domains 🧑🏫👩🏫. This allows us to offer well-rounded perspectives and knowledge to our readers.
Our blog originally focused on spirituality and metaphysics, but we’ve since expanded to cover a wide range of niches. Don’t worry—we continue to publish a lot of articles on spirituality! Frequently visit our blog to explore our diverse content and stay tuned for more insightful reads.